Support Vector Machines for Enzyme Function Prediction
Neetika Nath
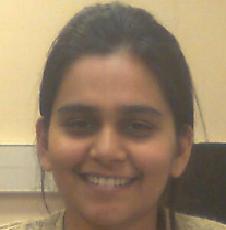
Our objective is to develop a computational method to predict an enzyme's reaction mechanism from its structure. Such a tool will have diverse applications including the modification and design of enzymes for use in diagnostics, biofuels, medicines, laundry, deodorants and many other products. When combined with protein structure prediction, it will also be valuable in the annotation of genomic sequence data.
Prediction of catalytic function from three dimensional structure remains an important problem, since structural genomics projects have generated structures of numerous proteins whose molecular functions have not been determined experimentally. For structures, and indeed for sequences, that are homologous to other well characterised proteins, tentative functional assignment is possible on that basis. Since distantly related enzymes are often found to have significantly different functions, however, a detailed comparison of active sites and of putative chemical reaction mechanisms is necessary for truly reliable predictions.
Support Vector Machine (SVM) is a powerful Machine Learning technique that involves mapping the data into a suitable high dimensional space in which a hyperplane can be found which separates instances belonging to different classes - here, enzymes with different functions. We will also compare the SVM results with those of other machine learning techniques, such as Random Forest. The data presented to the SVM will include the results of three dimensional template searches, and also of cross-docking ligands of other enzymes of known function into the query structure.
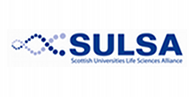
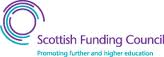